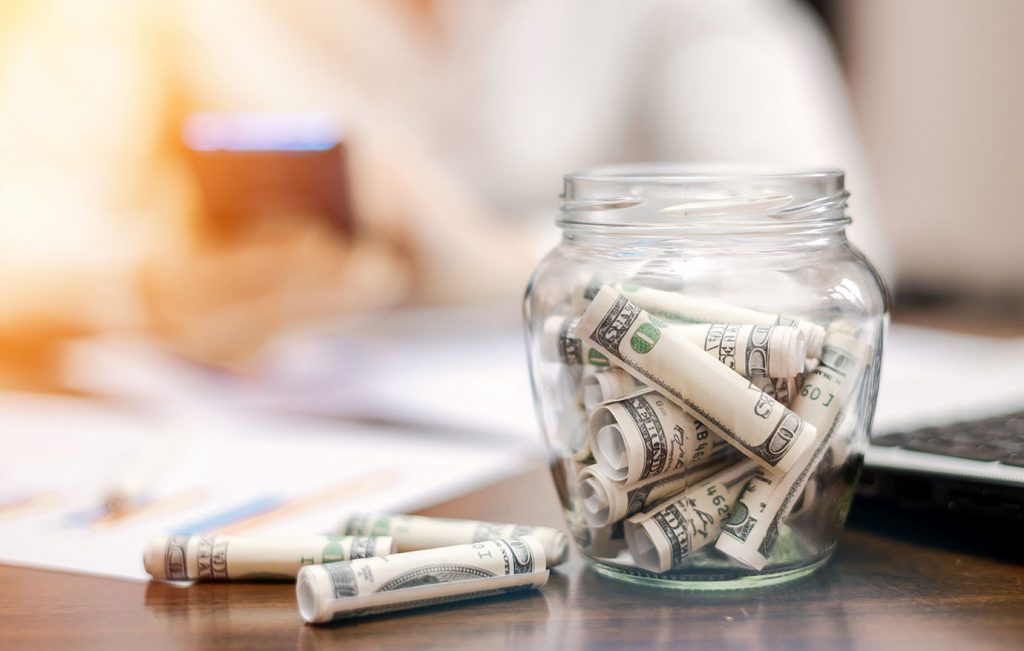
IoT Analytics released a research article that highlights 6 out of 17 industry trends included in the Embedded World 2024 Event Report.
This report presents key highlights and in-depth insights assembled by the IoT Analytics analyst team from one of the world’s leading fairs for the embedded community.
Key Insights:
The current state of embedded systems was on full display at Embedded World 2024, with a clear emphasis on edge AI. As part of the Embedded World 2024 Event Report, IoT Analytics’ team of four on-the-ground analysts identified 17 industry trends related to IoT chipsets and edge computing—this article highlights 6 of these trends related to edge AI.Key Quotes:
Satyajit Sinha, Principal Analyst at IoT Analytics, remarks:
“The shift towards edge AI will necessitate that CPU vendors develop not only high-performance multi-core CPUs but also integrate specialized NPUs into their SoC designs. The recent increase in demand for NVIDIA GPUs—driven by AI workloads—and the prevailing AI chip shortages have led to upward pressure on prices within the AI chipset market and could continue to do so for the foreseeable future.”
About Embedded World 2024
Embedded World is a leading event for the embedded systems community. This year, it took place from April 9 to April 11 in Nürnberg, Germany, and once again, it showcased the latest developments and innovations in embedded systems, embedded software, chipsets, edge computing, and related topics.
Attendance was up 19% from the previous year and has returned to pre-pandemic participation levels (~32,000 visitors). The number of vendors, too, returned to and even surpassed pre-pandemic levels, with a record 1,100.
IoT Analytics had a team of four analysts on the ground. They visited more than 60 booths and conducted over 35 individual interviews to comprehensively understand the most recent developments in embedded systems, with a special focus on IoT.
Embedded World 2024 emphasized the integration of AI within embedded systems, with a clear focus on edge AI. Corporate research subscribers can refer to the 67-page Embedded World 2024 Event Report for more information about the event, including highlights from keynote speeches, important announcements and launches, and major trends identified by the team. Here, the team shares only six of these trends, each based on observations about the future of edge AI.
To answer the question of what edge AI is, it is important to understand edge computing.
What is edge computing?
IoT Analytics defines edge computing as intelligent computational resources located close to the source of data consumption or generation. The edge includes all computational resources at or below the cell tower data center and/or on-premises data center, and there are 3 types of edges—thick, thin, and micro—as shown below.
What is edge AI?
Based on the above, edge AI is the deployment of AI models on a device or piece of equipment at the edge, thus enabling AI inference and decision-making without reliance on continuous cloud connectivity.
6 edge AI trends observed at Embedded World 2024
“Edge AI will reshape our world in a profound way.”
Edge AI was the key theme throughout the conference. Salil Raje, SVP of adaptive and embedded computing at AMD, best captured the energy around this topic during his keynote address, stating, “We stand on the brink of an era where edge AI will reshape our world in a profound way.”
On the stage, Salil Raje and Eiji Shibata, CDO at carmaker Subaru, discussed how AMD and Subaru are collaborating on an edge AI system for autonomous driving based only on cameras—with the vision to achieve zero accidents by 2030.
Below, the team highlights 6 trends it observed on the topic of edge AI.
1. NVIDIA becoming a key edge (AI) computing company
US-based chipmaker NVIDIA has played a crucial role in driving the adoption and implementation of AI technologies across various sectors. NVIDIA’s GPUs, renowned for their high-performance capabilities, specifically in data centers, are also becoming integral to deploying complex AI models at the edge. With a partner network of over 1,100 companies, NVIDIA has established a dominant position in the AI technology market, far ahead of its competitors AMD and Intel.
At Embedded World 2024, one such partner, Taiwan-based embedded systems provider Aetina, introduced its AI-driven industrial edge solutions powered by NVIDIA GPUs, such as its AIB-MX13/23, which is powered by NVIDIA’s Jetson AGX Orin GPU capable of 275 trillion or tera operations per second (TOPS). Using a portable ultrasonic testing device connected to the AIB-MX13/23, Aetina and its partner, Finland-based defect recognition solutions provider TrueFlaw, demonstrated a non-destructive evaluation method for fault detection.
Additionally, Taiwan-based fabless semiconductor company MediaTek showcased four new embedded systems-on-chips (SoCs) for automotive applications—CX-1, CY-1, CM-1, and CV-1—which support NVIDIA’s DRIVE OS 3 autonomous vehicle reference operating system. This application demonstrates how NVIDIA’s technologies are expanding into new domains beyond the gaming and data center GPUs they are generally known for.
2. Simplifying on-device AI inferencing processes for developers
The integration of on-device AI comes with various challenges. One key challenge that developers often face is the dilemma of investing in new devices before they can evaluate the performance of the AI chipset and its compatibility with an AI model. Evaluation factors for developers can include device TOPS, CPU/NPU percent utilization, and temperature. To solve this and other related problems, companies are launching new AI developer platforms that can simulate on-device AI performance, allowing developers to test AI model deployment using specific edge device/chipset resource specifications without purchasing the physical hardware.
One solution on display at Embedded World 2024 was Taiwan-based IoT and embedded solutions provider Advantech‘s EdgeAI SDK platform. This platform supports deploying AI models over widely recognized AI chipsets like Intel, NVIDIA, Qualcomm, and Hailo. Advantech showcased a pose detection model running on an AIMB-278 industrial motherboard integrated with Intel’s ARC A380E embedded systems GPU. Advantech’s EdgeAI SDK facilitated the model’s deployment.
3. AI model training shifting to the thick edge
AI model training is shifting from centralized cloud setups to thick-edge locations like servers or micro data centers. This is possible due to the integration of high-performance CPUs and GPUs that enable powerful computing at the edge, AI training, and multiple AI inferencing capabilities. Further, AI training can also happen on vendor premises, reducing reliance on cloud infrastructure, lowering costs, enhancing privacy, and improving the responsiveness of AI applications on edge devices.
Just before Embedded World 2024, US-based computer builder MAINGEAR and Taiwan-based memory controller manufacturer Phison announced the launch of MAINGEAR PRO AI workstations integrated with 4x NVIDIA’s RTX 5000 Ada or 4x RTX 6000 Ada GPUs with more than 1000 TFLOPS computing power.
At the event, Aetina launched its AIP-FR68 Edge AI Training platform, supporting various 4x NVIDIA GPUs with up to 200 teraflops—the number of float-point operations a chip can perform—of computing power, a lot for a single GPU.
4. Accelerating micro- and thin-edge AI through NPU integration
Integrating dedicated NPUs within edge devices greatly enhances AI inference capabilities. Additionally, it results in power savings, improved thermal management, and efficient multitasking, enabling the deployment of AI in power-sensitive and latency-critical applications, such as wearables and sensor nodes.
At the fair, the Netherlands-based semiconductor manufacturer NXP showcased its new MCX N Series MCUs, which provide 42 times faster ML inference than CPU cores alone. Additionally, UK-based semiconductor design company ARM demonstrated an ARM Cortex A55-only setup and an ARM Cortex A55 + ARM Ethos U65 NPU setup for AI inferencing. The latter setup offloaded 70% of AI inferencing from the CPU to the NPU, with an 11x improvement in inference performance.
5. Localizing autonomous decision-making via cellular-connected micro- and thin-edge AI
Integrating AI-enabled chipsets directly into cellular IoT devices is on the rise, marking a transformation toward intelligent, autonomous IoT systems capable of localized decision-making. This trend will likely substantially impact industries like smart cities and factories, and it brings significant advantages, including real-time data processing, reduced latency, and greater efficiency due to smaller form factors.
An example is the intelligent mowing robot solution displayed by China-based wireless communications module vendor Fibocom. It utilizes a Qualcomm-based intelligent module for powerful on-device computation, allowing it to not only map its environment and avoid obstacles but also perform cost-effective boundary recognition, all without constant reliance on the cloud. This practical application demonstrates the tangible value of AI-enabled chipsets in IoT devices.
Further, the US-based IoT solutions joint venture Thundercomm showcased its EB3G2 IoT edge gateway, which leverages a Qualcomm SoC for on-device AI model execution. This SoC enables immediate data analysis, reducing latency and cloud dependence. The gateway’s algorithms are capable of human detection and tracking, making it valuable for security and traffic management.
6. Tiny AI/ML bringing micro-edge AI capability to traditional devices
As the name suggests, tiny AI/ML are small-sized AI and ML models capable of running on resource-constrained devices, such as sensor-based micro-edge devices. The analyst team noted several cases of tiny ML being integrated into everyday objects and tools, enabling them to perform decision-making functions autonomously without the need for cloud connectivity. This approach bolsters privacy and data security by processing information directly on the device—at the very edge.
UK-based voice intelligence platform developer MY VOICE AI showcased NANOVOICE TM, a speaker verification solution powered by tiny ML and designed for ultra-low-power edge AI platforms. The solution combines passcode verification with speaker recognition for enhanced security.
Likewise, US-based AI/ML software company SensiML demonstrated a proof-of-concept for a smart drill that uses AI/ML models to classify different screw fastening states. The model is capable of both real-time edge sensing and anomaly detection. Further, Norway-based fabless semiconductor company Nordic Semiconductor showcased its Thingy53 IoT prototyping device embedded with Nordic’s nRF5340 chipset, which enables anomaly detection via embedded ML. When paired with an accelerometer, the Thingy:53 senses equipment vibrations using an embedded tiny ML model. As an example, this system could cut off power to a device or machine when it detects anomalies.
The future of the embedded world: what these edge AI trends mean for IoT embedded systems
Embedded World 2024 emphasized the growing role of edge AI within IoT systems. The developments the team witnessed focused on easier AI inferencing and a spectrum of edge AI solutions (micro, thin, and thick), pointing to greater intelligence at network edges.
Edge AI is shifting intelligent computation away from cloud-centric models and moving it closer to data sources. Driving this shift are reduced network traffic, near-instantaneous decision-making for time-critical applications (e.g., manufacturing, autonomous systems), and enhanced privacy by processing data locally. Ultimately, edge AI reduces reliance on hyperscalers and promotes broader AI usage outside centralized infrastructure. It holds transformative potential across healthcare, automotive, and robotics, with the capability to reshape operational paradigms within these industries.
Looking ahead, edge AI will have varying impacts across edge levels:
Thick edge AI: Facilitate the execution of multiple AI inference models on edge servers or at the network periphery and support AI model training or retraining for scenarios involving sensitive data on premises Thin edge AI: Enhance the intelligence of existing sensors and devices by utilizing gateways, IPCs, and PLCs for AI processing at the network edge Micro edge: Enable direct AI integration into sensors, improve the scalability of intelligent systems, and empower everyday connected devices to make autonomous decisionsThe post The top 6 edge AI trends – as showcased at Embedded World 2024 appeared first on IoT Business News.
More Stories
Berg Insight unveils comprehensive research on the cellular IoT antenna market
Soracom IoT Platform Achieves SOC 2 Type 1 Compliance for Internal Control
Internet of Things in Retail Market Size Will Expand to USD 464.9 Billion by 2031